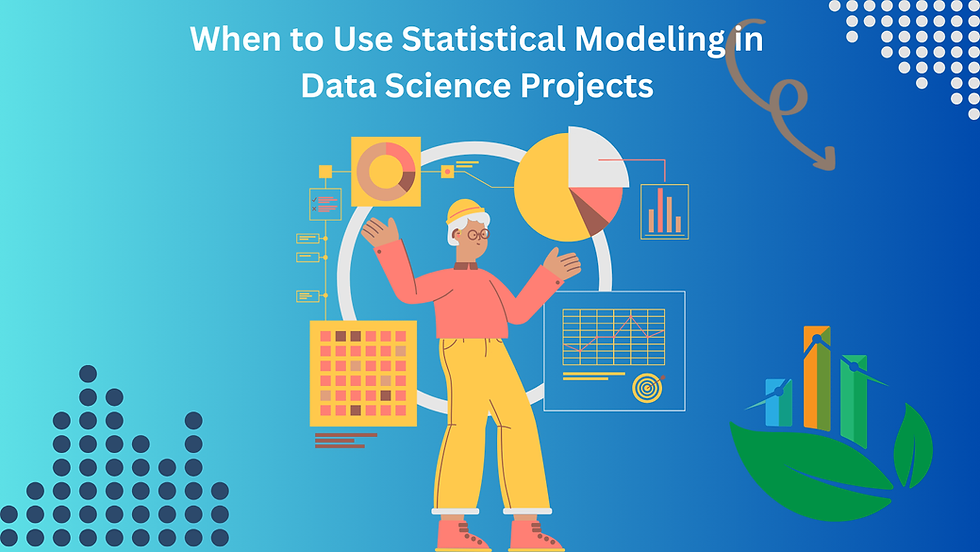
In the realm of data science, statistical modeling plays a crucial role in understanding and interpreting complex data. It provides a framework for making predictions, identifying patterns, and drawing meaningful conclusions from data. However, knowing when to employ statistical modeling can be challenging. This article explores key scenarios where statistical modeling is beneficial, guiding you in making informed decisions in your data science projects.
Understanding Statistical Modeling
Before diving into specific use cases, it's essential to understand what statistical modeling entails. At its core, statistical modeling involves creating mathematical representations of real-world processes using data. These models help you estimate relationships between variables, predict future outcomes, and test hypotheses.
Statistical models can range from simple linear regressions to more complex machine learning algorithms. The choice of model depends on the nature of your data and the questions you seek to answer.
When to Use Statistical Modeling
1. When You Need to Make Predictions
One of the primary reasons to use statistical modeling is to make predictions based on historical data. If you have a dataset and want to forecast future trends, statistical models can help. For example:
Sales Forecasting: Businesses often use statistical models to predict future sales based on past performance. Techniques like time series analysis can uncover trends and seasonality, helping organizations make informed inventory and marketing decisions.
Customer Churn Prediction: Companies can use statistical models to identify which customers are likely to stop using their services. By analyzing historical data, businesses can develop focused retention strategies.
2. When You Want to Identify Relationships
Statistical modeling is also valuable for exploring relationships between variables. Understanding these relationships can provide insights that drive strategic decisions. For example:
Marketing Effectiveness: If you're running multiple marketing campaigns, statistical modeling can help you determine which strategies yield the best return on investment (ROI). By analyzing the relationship between ad spend and sales, you can optimize your marketing budget.
Health Outcomes: In healthcare, researchers often use statistical models to examine how different factors affect patient outcomes. For instance, they might explore the relationship between lifestyle choices and the likelihood of developing certain diseases.
3. When You're Testing Hypotheses
Statistical modeling is a powerful tool for hypothesis testing. If you have a specific question or theory, statistical models can help you validate or refute your assumptions. For example:
A/B Testing: In the tech industry, A/B testing is common for optimizing user experiences. By applying statistical models to compare two versions of a product, you can determine which performs better and make data-driven decisions.
Social Science Research: Researchers in social sciences often use statistical models to test hypotheses about human behavior. For example, they might analyze survey data to see if there's a significant relationship between education level and job satisfaction.
4. When You're Working with Complex Data
Statistical modeling is especially useful when dealing with large and complex datasets. Techniques such as multivariate regression can handle multiple variables simultaneously, helping you make sense of intricate relationships. For example:
Finance: In the financial sector, analysts use statistical models to assess risk and return by considering various factors, such as market conditions, interest rates, and economic indicators.
Climate Science: Climate researchers rely on statistical models to understand the interactions between different climate variables. These models help predict future climate patterns and inform policy decisions.
5. When Data is Noisy or Incomplete
Real-world data is often messy, with missing values or noise. Statistical modeling can help you draw meaningful conclusions despite these imperfections. For instance:
Imputation Techniques: Statistical models can estimate missing values based on the patterns observed in the data, allowing for more robust analysis.
Robustness to Outliers: Some statistical models, like robust regression, are designed to minimize the impact of outliers, providing more reliable results in the presence of noisy data.
6. When You Need to Communicate Insights
Statistical models not only provide results but also facilitate effective communication of insights. By using visualizations and statistical summaries, you can convey complex findings to stakeholders who may not be data-savvy. For example:
Dashboards: Creating interactive dashboards that display key metrics derived from statistical models can help stakeholders quickly grasp important trends and make data-driven decisions.
Reports: When presenting findings to non-technical audiences, statistical models can help simplify complex information, making it accessible and actionable.
Conclusion
Statistical modeling is a powerful tool in the data scientist's toolkit, particularly for those enrolled in a Data Science Training Course in Delhi, Noida, Lucknow, Nagpur, and other cities in India. Knowing when to use it can significantly enhance the effectiveness of your data science projects. Whether you're making predictions, exploring relationships, testing hypotheses, or working with complex data, statistical models can provide valuable insights that drive informed decisions.
As you embark on your data science journey, especially through a comprehensive training course, remember that the key to successful statistical modeling lies in understanding your data, the questions you want to answer, and the context in which you're working. With careful consideration, you can leverage statistical modeling to unlock the full potential of your data and make the most of your training experience.
Comments