When to Use Data Science for Problem Solving: A Simple Guide
- k86874248
- Sep 12, 2024
- 4 min read
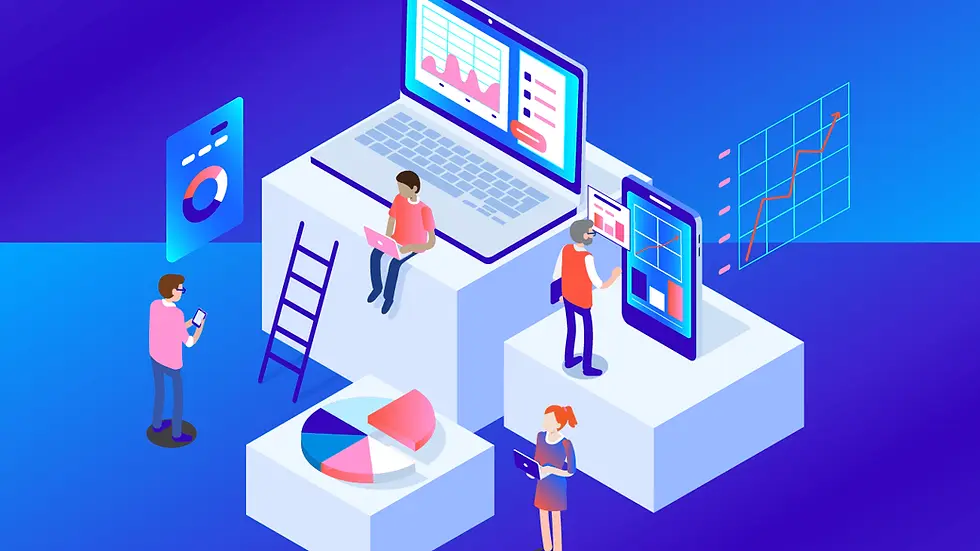
In today’s world, data is everywhere. We have more information than ever before, and this can be overwhelming. However, with the right tools and techniques, this data can be transformed into valuable insights. Data science is one such tool that helps us make sense of large amounts of information. But when should you use data science to solve a problem? Let’s break it down into easy-to-understand terms.
What is Data Science?
Before diving into when to use data science, let’s clarify what it is. Data science involves collecting, analyzing, and interpreting complex data to help make informed decisions. It combines elements from statistics, computer science, and domain knowledge to uncover patterns and insights from data.
Signs You Might Need Data Science
Complex Data Sets:
Example: You’re running an e-commerce store and have millions of customer transactions. The data includes purchase histories, customer demographics, and browsing behaviors. This data is too complex to analyze manually.
When to Use Data Science: When you have large volumes of data that can’t be easily processed or analyzed using simple tools. Data science can help find patterns and trends that might not be obvious at first glance.
Need for Predictive Insights:
Example: You want to forecast sales for the next quarter based on historical data, seasonal trends, and market conditions. Predicting future trends can be challenging without the right tools.
When to Use Data Science: When you need to make predictions about future events based on historical data. Data science uses statistical models and machine learning algorithms to forecast outcomes and trends.
Complex Decision-Making:
Example: You’re a city planner needing to decide where to build a new park. You have data on population density, traffic patterns, and existing amenities. Making an informed decision requires considering multiple factors.
When to Use Data Science: When decisions are complex and require analyzing multiple variables and their interactions. Data science helps in weighing different factors and making data-driven decisions.
Understanding Customer Behavior:
Example: You want to understand why certain customers are abandoning their shopping carts on your website. You have data on user interactions, but you need insights to improve conversion rates.
When to Use Data Science: When you need to analyze customer behavior and preferences. Data science can help segment customers, identify patterns, and understand what influences their decisions.
Identifying Anomalies:
Example: You notice an unusual spike in your company’s expenses. Manual checks aren’t revealing the cause. Anomaly detection can help identify irregular patterns.
When to Use Data Science: When you need to detect unusual patterns or outliers in your data. Data science techniques can identify anomalies that might indicate fraud, errors, or other issues.
Optimizing Processes:
Example: Your manufacturing process has inefficiencies, but you’re unsure where the problem lies. Data science can analyze various metrics to pinpoint the issue.
When to Use Data Science: When you need to optimize processes and improve efficiency. Data science can analyze operational data to identify bottlenecks and suggest improvements.
How Data Science Solves Problems
Here’s a step-by-step look at how data science can be applied to problem-solving:
Define the Problem:
Clearly articulate what you want to solve. The problem should be specific and actionable. For instance, “Why are our customer churn rates increasing?” is a clear problem statement.
Collect and Prepare Data:
Gather the relevant data needed to address the problem. This might involve cleaning and organizing data to make it suitable for analysis.
Explore the Data:
Use exploratory data analysis (EDA) to understand the data’s structure and relationships. This step helps in identifying patterns, trends, and potential issues.
Apply Data Science Techniques:
Depending on the problem, use appropriate data science methods such as statistical analysis, machine learning, or predictive modeling. For example, if you’re predicting future sales, you might use regression models.
Interpret the Results:
Translate the data findings into actionable insights. Ensure that the results are understandable and relevant to the problem.
Make Data-Driven Decisions:
Use the insights gained to make informed decisions. For instance, if data analysis reveals that certain marketing strategies are more effective, you can focus your efforts on those strategies.
Monitor and Iterate:
After implementing changes based on data insights, monitor the outcomes. Data science is an iterative process, and continuous monitoring helps in refining solutions and making further improvements.
When Not to Use Data Science
While data science is powerful, it’s not always the best tool for every problem. Here are some scenarios where data science might not be necessary:
Simple Problems with Clear Solutions:
Example: If you need to calculate the total cost of ingredients for a recipe, a simple calculation suffices. Data science would be overkill here.
Lack of Data:
Example: If there’s no available data on customer satisfaction, data science cannot help. It relies on having data to analyze.
Short-Term or Intuitive Decisions:
Example: For decisions that don’t require extensive analysis and are based on intuition or immediate feedback, data science might not be needed.
Conclusion
Data science is a powerful tool for solving complex problems, making predictions, and gaining insights from data. It’s particularly useful when dealing with large data sets, complex decision-making, or when you need to understand customer behavior. For those looking to deepen their knowledge, enrolling in a Data Science course in Lucknow, Nagpur, Delhi, Noida, or other cities in India can provide essential expertise and hands-on experience.
However, it’s important to recognize when data science is not necessary and to use simpler methods when appropriate. By understanding when and how to apply data science, you can leverage it effectively to make informed decisions, optimize processes, and achieve better outcomes. Whether you’re a business owner, a researcher, or someone interested in data, knowing how to strategically use data science can be a game-changer in solving your problems.
Comments