Time Series Analysis: Methods and Applications
- k86874248
- Jul 9, 2024
- 3 min read
Updated: Feb 21
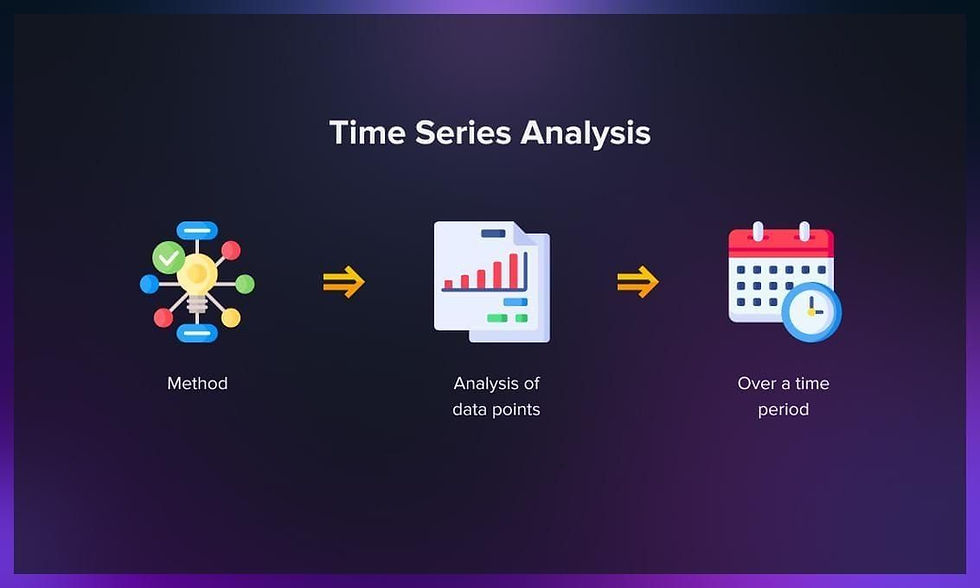
Time series analysis involves examining datasets over a period of time to identify trends, patterns, and seasonal variations. It is widely used in various fields, including finance, economics, environmental science, and healthcare. Understanding the fundamental concepts, methods, and applications of time series analysis can help you leverage this powerful tool for making informed decisions.
Fundamental Concepts
Trend: The long-term movement in a time series. It shows the general direction of the data over a period of time.
Seasonality: The repeating short-term cycle in a time series. It highlights the regular pattern of fluctuations within a specific period, such as monthly or quarterly.
Cyclical Component: These are fluctuations occurring at irregular intervals due to economic conditions, often spanning several years.
Irregular Component: The residual variation in a time series after removing trend, seasonality, and cyclical components. It is random and unpredictable.
Stationarity is essential for many time series forecasting models to perform accurately.
Methods of Time Series Analysis
Plotting: Visualizing data through line plots, scatter plots, and histograms helps identify trends, seasonality, and outliers.
Summary Statistics: Calculating mean, median, standard deviation, and other statistics to summarize the data.
Moving Average: A technique used to smooth out short-term fluctuations and highlight long-term trends or cycles.
AR (Autoregressive) Component: A model that uses the dependency between an observation and a number of lagged observations.
I (Integrated) Component: Represents the differencing of raw observations to make the time series stationary.
MA (Moving Average) Component: A model that uses the dependency between an observation and a residual error from a moving average model applied to lagged observations.
Extends ARIMA by explicitly modeling seasonal components. It includes seasonal autoregressive, seasonal differencing, and seasonal moving average terms.
ARCH: A model used for time series data that exhibit time-varying volatility clustering, such as financial time series.
GARCH: Extends ARCH by modeling both the autoregressive and moving average components of the variance.
Applications of Time Series Analysis
Stock Market Analysis: Forecasting stock prices and indices, identifying trends and cycles, and managing risks.
Economic Forecasting: Predicting economic indicators like GDP, unemployment rates, and inflation.
Climate Studies: Analyzing temperature, precipitation, and other climate-related data to identify trends and changes.
Environmental Monitoring: Tracking pollution levels, deforestation rates, and other environmental metrics.
Epidemiology: Tracking the spread of diseases, predicting outbreaks, and evaluating the effectiveness of interventions.
Patient Monitoring: Analyzing vital signs data to detect anomalies and predict patient outcomes.
Demand Forecasting: Predicting future product demand to optimize inventory levels and reduce stockouts.
Sales Trend Analysis: Identifying sales trends and seasonality to develop effective marketing strategies.
Load Forecasting: Predicting electricity demand to ensure reliable power supply and optimize energy production.
Renewable Energy: Analyzing data from wind turbines, solar panels, and other renewable energy sources to optimize performance and maintenance.
Practical Steps for Time Series Analysis
Gather relevant time series data from reliable sources. Ensure the data is of high quality, with minimal missing values and outliers.
Handle missing values through imputation or interpolation.
Remove outliers or correct anomalies that can skew the analysis.
Transform non-stationary data into a stationary form through differencing or other techniques.
Choose the appropriate model based on the data characteristics and the analysis objectives. ARIMA and SARIMA models are suitable for univariate time series, while multivariate time series may require more complex models.
Split the data into training and testing sets. Fit the model to the training data and validate it using the testing data.
Evaluate the model's performance using metrics such as Mean Absolute Error (MAE), Mean Squared Error (MSE), and Root Mean Squared Error (RMSE).
Use the fitted model to make forecasts. Interpret the results in the context of the application and use them to inform decision-making.
Conclusion
Time series analysis is a versatile tool with numerous applications across various fields. By understanding the fundamental concepts and methods, you can effectively analyze time series data, uncover hidden patterns, and make accurate forecasts. Whether you're working in finance, healthcare, environmental science, or any other domain, time series analysis can provide valuable insights to drive better decisions. This guide provides an overview of the essential methods and applications of time series analysis. For those interested in mastering these skills, a Data Analytics course in Nagpur, Lucknow, Delhi, Noida, and all locations in India can provide comprehensive training and resources. By applying these techniques, you can harness the power of time series data to address real-world challenges and opportunities.
Comentários