Time Series Analysis: Methods and Applications
- k86874248
- Jun 20, 2024
- 3 min read
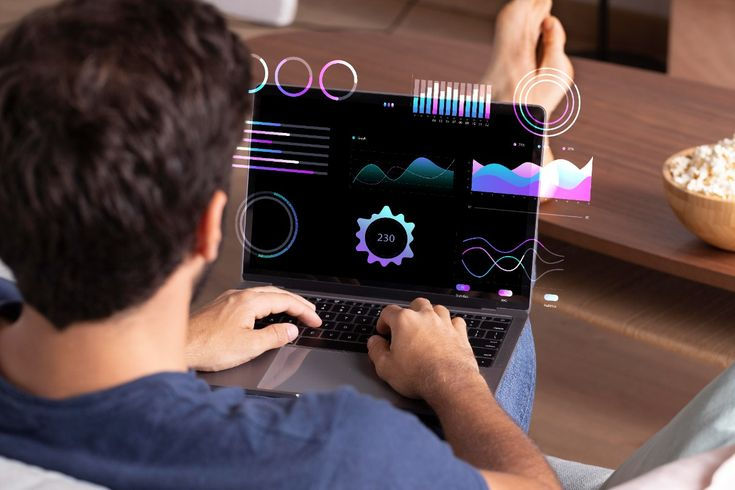
Introduction
Time series analysis involves studying datasets composed of sequentially recorded data points, typically measured over regular intervals. This type of analysis helps in identifying patterns, trends, and potential future behaviors based on historical data. From finance and economics to weather forecasting and supply chain management, time series analysis is a crucial tool for data-driven decision-making.
Basic Concepts
Time Series Components
Trend: The long-term movement in the data. It shows the general direction, either upward or downward, over a significant period.
Seasonality: Regular patterns that repeat at fixed intervals, such as monthly or quarterly sales.
Cyclic Patterns: Longer-term fluctuations related to economic cycles or other external factors, which are less predictable than seasonal patterns.
Irregular Components: Random noise or residuals that are not explained by the trend, seasonality, or cyclic components.
Methods of Time Series Analysis
1. Descriptive Analysis
Descriptive analysis summarizes the main features of a dataset, providing simple summaries and visualizations. Common techniques include:
Line Plots: Visual representations of data points over time.
Summary Statistics: Measures such as mean, median, variance, and standard deviation.
2. Decomposition
Decomposition involves breaking down a time series into its constituent components: trend, seasonality, and residuals. This method helps in understanding underlying patterns and is typically performed using:
Additive Model: Assumes that components add together (useful when seasonal variations are roughly constant).
Multiplicative Model: Assumes components multiply together (useful when seasonal variations change proportionally with the level of the time series).
3. Smoothing Techniques
Smoothing techniques are used to remove noise and highlight the underlying patterns:
Moving Averages: Calculates the average of different subsets of the complete dataset to smooth out short-term fluctuations.
Exponential Smoothing: Applies decreasing weights to past observations to smooth data. There are several types, including Single, Double, and Triple Exponential Smoothing (Holt-Winters).
4. Autoregressive Integrated Moving Average (ARIMA)
ARIMA models are widely used for forecasting. The model is characterized by three parameters:
Autoregressive (AR) part: Indicates that the evolving variable of interest is regressed on its own prior values.
Integrated (I) part: Represents the differencing of raw observations to make the time series stationary.
Moving Average (MA) part: Represents the dependency between an observation and a residual error from a moving average model applied to lagged observations.
5. Seasonal ARIMA (SARIMA)
SARIMA extends ARIMA by explicitly modeling seasonal effects. This is useful when the time series shows regular seasonal patterns.
Applications of Time Series Analysis
1. Finance
Time series analysis is extensively used in finance for:
Stock Price Prediction: Modeling and forecasting stock prices and indices.
Risk Management: Analyzing historical data to predict future risks and manage portfolios.
Economic Forecasting: Predicting economic indicators like GDP, inflation rates, and unemployment.
2. Weather Forecasting
Meteorologists use time series analysis to predict weather patterns, temperature trends, and precipitation levels, relying on historical data to identify cycles and trends.
3. Supply Chain Management
In supply chain management, time series analysis helps in:
Demand Forecasting: Predicting future product demand to optimize inventory levels.
Production Planning: Scheduling production based on expected demand patterns.
4. Healthcare
Healthcare professionals use time series analysis to:
Monitor Patient Health: Analyzing vital signs over time to detect anomalies or trends.
Disease Outbreak Prediction: Forecasting the spread of diseases based on historical infection rates.
5. Marketing
Marketers use time series analysis for:
Sales Forecasting: Predicting future sales to plan marketing strategies.
Customer Behavior Analysis: Understanding how customer preferences change over time.
Conclusion
Time series analysis offers valuable insights by examining data points collected over time. By identifying patterns, trends, and potential future movements, businesses and researchers can make informed decisions in various fields, from finance and weather forecasting to healthcare and marketing. Understanding and applying the right methods and models is crucial for leveraging the full potential of time series data. For those looking to enhance their skills in this area, a Data Analytics course in Lucknow, Gwalior, Delhi, Noida, and all locations in India can provide the necessary knowledge and expertise.
Comments