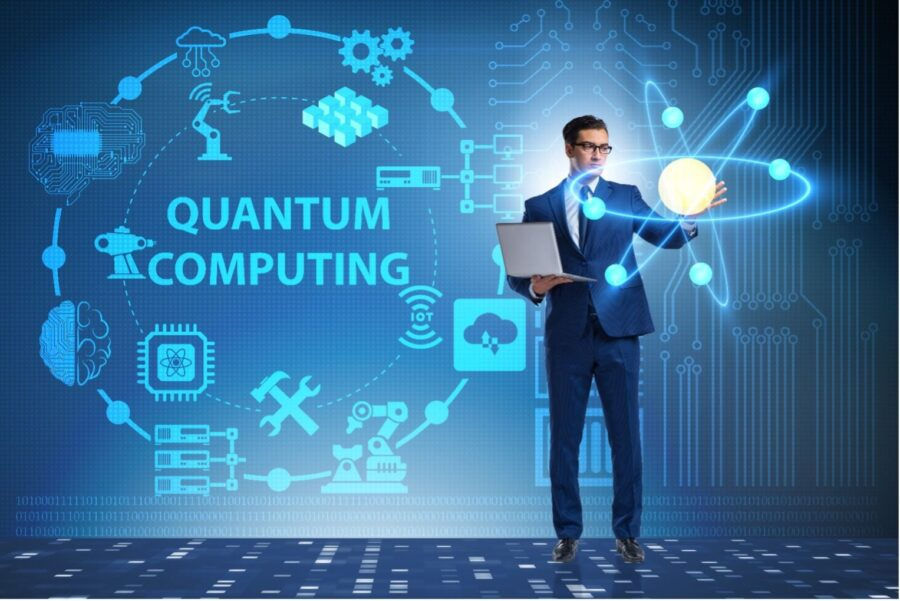
In recent years, quantum computing has become a buzzword in the tech world, and for good reason. It's a cutting-edge technology that promises to revolutionize many fields, including data science. But what exactly is quantum computing, and how could it impact the world of data science? Let’s break it down in simple terms.
What is Quantum Computing?
To understand quantum computing, it's helpful to first grasp the basics of classical computing. Classical computers, like the one you use daily, process information using bits. These bits are like tiny switches that can either be off (0) or on (1). By combining billions of these bits, computers can perform complex calculations and tasks.
Quantum computers, on the other hand, use quantum bits or qubits. Unlike classical bits, qubits can be both 0 and 1 at the same time, thanks to a principle called superposition. This means quantum computers can process a vast amount of possibilities simultaneously.
Another key principle is entanglement. When qubits become entangled, the state of one qubit is linked to the state of another, regardless of the distance between them. This allows quantum computers to solve certain problems much more efficiently than classical computers.
Data Science: The Basics
Data science is the field that uses scientific methods, algorithms, and systems to extract knowledge and insights from data. It's a multidisciplinary field that combines statistics, computer science, and domain expertise to analyze and interpret large sets of data.
Key tasks in data science include:
Data Collection: Gathering data from various sources.
Data Cleaning: Removing or correcting errors and inconsistencies in the data.
Data Analysis: Applying statistical methods to understand and summarize the data.
Predictive Modeling: Using algorithms to make predictions based on historical data.
Data Visualization: Creating charts and graphs to make data insights understandable.
How Quantum Computing Could Transform Data Science
Quantum computing could significantly impact data science in several ways:
Enhanced Data Processing Speed One of the biggest advantages of quantum computing is its potential to process data much faster than classical computers. Traditional data processing involves complex calculations that can take a long time, especially as data volumes grow. Quantum computers, with their ability to handle multiple possibilities at once, could speed up these calculations dramatically.For instance, a quantum computer could quickly solve optimization problems that involve finding the best solution from a vast number of possibilities. This capability could revolutionize industries that rely heavily on optimization, such as finance and logistics.
Improved Predictive Analytics Predictive analytics is a crucial aspect of data science, used to forecast future trends based on historical data. Quantum computing could enhance predictive models by processing and analyzing more complex datasets more efficiently. For example, it could help in developing more accurate models for stock market predictions, weather forecasting, or customer behavior analysis.Quantum algorithms, like the Quantum Fourier Transform, can perform certain types of computations exponentially faster than classical algorithms, potentially leading to breakthroughs in predictive analytics.
Better Handling of Big Data The volume of data generated today is enormous, and managing and analyzing this big data can be challenging. Quantum computing's ability to process large datasets efficiently could address some of these challenges. Quantum algorithms could potentially sift through massive amounts of data to identify patterns and insights that classical computers might miss.For instance, quantum computers could enhance machine learning algorithms, enabling them to learn from bigger datasets and make more accurate predictions. This could lead to advancements in fields such as personalized medicine, where large datasets are used to tailor treatments to individual patients.
Advanced Machine Learning Machine learning is a subset of data science that focuses on building algorithms that allow computers to learn from and make decisions based on data. Quantum computing could take machine learning to the next level by enabling more complex models and faster training times.Quantum algorithms like Quantum Support Vector Machines or Quantum Neural Networks could offer new ways to approach machine learning problems. These algorithms could potentially handle higher-dimensional data and find more accurate solutions to complex problems.
Enhanced Optimization Problems Many data science tasks involve optimization problems, where the goal is to find the best solution from a set of possibilities. Quantum computing could provide significant improvements in solving these problems. For example, optimizing supply chain logistics or financial portfolios could benefit from quantum computing’s ability to evaluate multiple scenarios quickly.Quantum algorithms such as the Quantum Approximate Optimization Algorithm (QAOA) are designed to address these kinds of problems more efficiently than classical methods.
Challenges and Future Outlook
While quantum computing holds great promise, it's still in its early stages. There are several challenges that need to be addressed before it can become a mainstream tool in data science:
Technical Hurdles: Building and maintaining stable quantum computers is a complex task. Current quantum computers are still relatively small and prone to errors, which limits their practical use.
Cost: Quantum computing technology is expensive, and access to quantum computers is currently limited to a few research institutions and large companies.
Algorithm Development: Quantum algorithms are different from classical ones, and developing effective quantum algorithms for data science applications is an ongoing area of research.
Despite these challenges, researchers and tech companies are making significant progress. Major players like Google, IBM, and Microsoft are investing heavily in quantum computing, and new developments are emerging regularly. As the technology matures, we can expect to see more practical applications and breakthroughs in data science.
Conclusion
Quantum computing has the potential to revolutionize data science by enhancing data processing speeds, improving predictive analytics, and better handling big data. While the technology is still developing, its impact on the field could be profound, leading to more accurate models, faster insights, and new discoveries.
As quantum computing continues to evolve, it will be exciting to see how it transforms data science and opens up new possibilities for solving complex problems. For now, staying informed about these advancements and pursuing relevant education, such as Data Science Course in Nagpur, Lucknow, Delhi, Noida, and beyond, can help professionals and enthusiasts prepare for a future where quantum computing plays a significant role in their work.
Comments