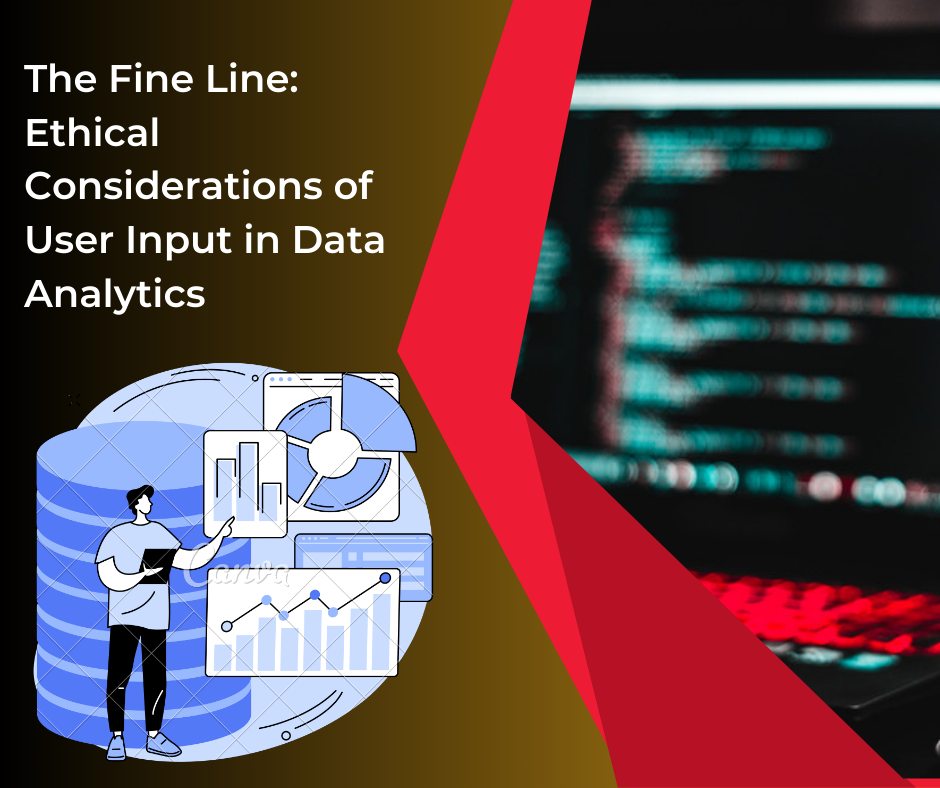
In today's data-driven world, the role of user input in data analytics is more significant than ever. Businesses and organizations increasingly rely on user-generated data to inform decisions, personalize services, and enhance customer experiences. However, this reliance brings forth critical ethical considerations that must be addressed to maintain trust, privacy, and fairness. This article explores these ethical considerations, highlighting the fine line data analysts must walk to balance innovation with integrity.
1. Privacy and Consent
Privacy is the cornerstone of ethical data analytics. Users often provide personal information, either knowingly or unknowingly, which can be used to infer sensitive details about their lives. Ensuring that user data is handled with the utmost care is paramount.
Informed Consent is a fundamental aspect of respecting user privacy. Users must be clearly informed about what data is being collected, how it will be used, and who will have access to it. Consent should be explicit and not buried in lengthy terms and conditions. Moreover, users should have the option to withdraw their consent at any time, and their data should be deleted upon request.
2. Data Security
Data Security involves protecting user data from unauthorized access, breaches, and misuse. Robust security measures, such as encryption, secure storage, and regular security audits, are essential. Data breaches not only violate user privacy but can also lead to identity theft, financial loss, and a significant erosion of trust.
3. Anonymization and De-identification
To mitigate privacy risks, data analysts often use anonymization and de-identification techniques. Anonymization involves altering data so that individuals cannot be identified, while de-identification removes or obscures personal identifiers. However, these methods are not foolproof. Re-identification can occur if anonymized data is combined with other data sources. Thus, ongoing vigilance and advanced techniques are required to ensure true anonymity.
4. Bias and Fairness
Bias in data analytics can lead to unfair and discriminatory outcomes. Bias can enter the data analytics process through biased data collection, algorithmic bias, or biased interpretation of results. For example, if a dataset used to train a predictive model lacks diversity, the model's predictions may be biased against certain groups.
Ensuring Fairness involves implementing measures to detect and mitigate bias at every stage of the data analytics process. This includes using diverse and representative datasets, regularly testing models for bias, and employing fairness-aware algorithms. Transparency in how data is collected and analyzed also plays a crucial role in maintaining fairness.
5. Transparency and Accountability
Transparency in data analytics means that organizations should be open about their data practices. Users have a right to know how their data is being used, what decisions are being made based on it, and what algorithms are involved. Transparency fosters trust and allows users to make informed choices about their data.
Accountability involves holding organizations and individuals responsible for ethical lapses in data analytics. This includes establishing clear guidelines and standards for data practices, as well as mechanisms for reporting and addressing unethical behavior. Regulatory frameworks, such as the General Data Protection Regulation (GDPR) in Europe, play a critical role in enforcing accountability.
6. Purpose Limitation and Data Minimization
Purpose Limitation requires that data be collected for specific, legitimate purposes and not used beyond those purposes without additional consent. This principle helps prevent misuse of data and respects the user's original intent.
Data Minimization involves collecting only the data that is necessary for the intended purpose. Excessive data collection increases privacy risks and can lead to data hoarding, where organizations keep more data than they need, simply because they might find a use for it later.
7. Ethical Use of Algorithms
Algorithms are the engines of data analytics, but their power must be wielded responsibly. The Ethical Use of Algorithms involves ensuring that they are designed and used in ways that respect user rights and promote fairness. This includes:
Algorithmic Transparency: Making the workings of algorithms understandable to non-experts.
Accountability: Being able to explain and justify the decisions made by algorithms.
Non-Discrimination: Ensuring algorithms do not perpetuate or exacerbate biases.
Responsibility: Regularly monitoring and updating algorithms to address any emerging ethical concerns.
8. User Empowerment and Control
Empowering users to control their own data is a crucial ethical consideration. This includes:
Access: Allowing users to access their own data and understand how it is being used.
Correction: Enabling users to correct any inaccuracies in their data.
Portability: Providing users with the ability to move their data between different services.
Deletion: Offering users the option to delete their data if they no longer want it to be used.
9. Long-term Implications and Societal Impact
Ethical data analytics also involves considering the Long-term Implications and Societal Impact of data practices. This means looking beyond immediate outcomes and considering how data use affects society as a whole. Issues such as the digital divide, where unequal access to data and technology can exacerbate social inequalities, must be addressed.
Conclusion
Navigating the ethical landscape of user input in data analytics is a complex but essential task. Privacy, consent, security, and fairness are just some of the critical issues that data analysts must consider. By adopting ethical practices and maintaining a user-centered approach, organizations can harness the power of data while respecting the rights and dignity of individuals. This balance is not only crucial for maintaining trust but also for fostering a fair and equitable data-driven society. For those interested in mastering these ethical considerations, enrolling in a Data Analytics course in Gwalior, Lucknow, Delhi, Noida, and all locations in India can provide the necessary knowledge and skills to navigate this complex field effectively.
Comments