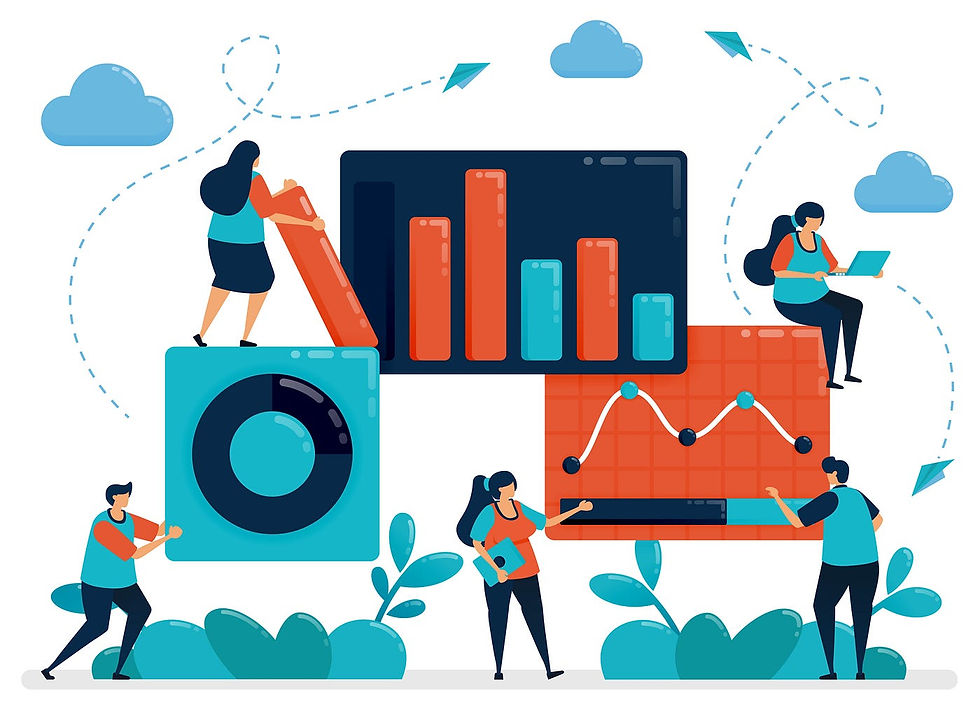
In our data-driven world, statistics is essential for interpreting and understanding data. Whether you are just starting in data science or have years of experience, a solid grasp of statistical fundamentals will enhance your ability to make informed decisions. This article offers a comprehensive overview of key statistical concepts critical for data science, presented in a clear and accessible manner.
1. Understanding Data Types
Before engaging in statistical analysis, it's crucial to identify the types of data you will encounter. Data is typically classified into two broad categories:
Quantitative Data
Quantitative data consists of numerical values and can be divided into two subtypes:
Discrete Data: These are countable values, such as the number of visitors to a website.
Continuous Data: This includes measurable quantities, like height, weight, or temperature.
Qualitative Data
Also referred to as categorical data, qualitative data comprises non-numeric values and can be classified into:
Nominal Data: Categories without a specific order (e.g., types of fruits or colors).
Ordinal Data: Categories with a defined order but no consistent differences between values (e.g., levels of customer satisfaction).
Recognizing these data types is vital for selecting the appropriate statistical techniques and tools.
2. Descriptive Statistics
Descriptive statistics summarize and describe the main features of a dataset. Here are some commonly used measures:
Measures of Central Tendency
Mean: The average value of a set of numbers.
Median: The middle value when the data is arranged in ascending order.
Mode: The most frequently occurring value in a dataset.
Measures of Dispersion
Range: The difference between the highest and lowest values in the dataset.
Variance: A measure of how far a set of numbers is spread out from the mean.
Standard Deviation: The square root of the variance, indicating how much individual values differ from the average.
These descriptive statistics provide a concise summary of the data, highlighting its distribution and variability.
3. Inferential Statistics
While descriptive statistics focus on summarizing data, inferential statistics enable us to make predictions and generalizations about a population based on a sample. Key concepts include:
Sampling
When studying an entire population is impractical, researchers select samples. A well-chosen sample can offer insights that are representative of the whole population.
Hypothesis Testing
Hypothesis testing involves making an assumption (the hypothesis) about a population parameter and using statistical methods to determine whether to accept or reject that hypothesis. Key elements include:
Null Hypothesis (H0): The assumption that there is no effect or difference.
Alternative Hypothesis (H1): The assumption that there is an effect or difference.
p-value: A measure that helps determine the significance of your results. A p-value below a predetermined threshold (often 0.05) indicates strong evidence against the null hypothesis.
Confidence Intervals
A confidence interval provides an estimated range of values likely to include the population parameter, offering an indication of the reliability of the estimate.
4. Correlation and Regression
Understanding relationships between variables is vital in data science. Two essential techniques are correlation and regression.
Correlation
Correlation assesses the strength and direction of a relationship between two variables, represented by the correlation coefficient (r), which ranges from -1 to 1:
Positive Correlation (r > 0): As one variable increases, the other also tends to increase.
Negative Correlation (r < 0): As one variable increases, the other tends to decrease.
No Correlation (r = 0): There is no apparent relationship between the two variables.
Regression
Regression analysis predicts the value of a dependent variable based on one or more independent variables. The simplest form is linear regression, which fits a straight line to the data points.
The linear regression equation is typically expressed as: Y=a+bXY = a + bXY=a+bX where:
YYY is the dependent variable,
aaa is the y-intercept,
bbb is the slope of the line, and
XXX is the independent variable.
5. Data Visualization
Visualizing data is crucial for effectively interpreting statistical results. Charts and graphs help convey complex information clearly. Common visualization techniques include:
Histograms: Useful for showing the distribution of a single variable.
Scatter Plots: Ideal for displaying the relationship between two quantitative variables.
Box Plots: Provide a summary of a dataset’s minimum, first quartile, median, third quartile, and maximum values.
Using appropriate visualizations can significantly enhance your ability to communicate findings and insights.
6. Conclusion
A solid understanding of statistics is essential for anyone involved in data science, and you can gain this knowledge through a comprehensive Data Science Training Course in Delhi, Noida, Lucknow, and more cities in India. By familiarizing yourself with data types, descriptive and inferential statistics, correlation and regression, and the importance of data visualization, you will improve your analytical skills and make well-informed decisions.
As you progress in your data science journey, remember that the goal of statistics is not just to process numbers but to extract meaningful insights that can drive decisions and strategies.
Comments