Probability Theory and Its Applications in Data Analytics
- k86874248
- Sep 23, 2024
- 3 min read
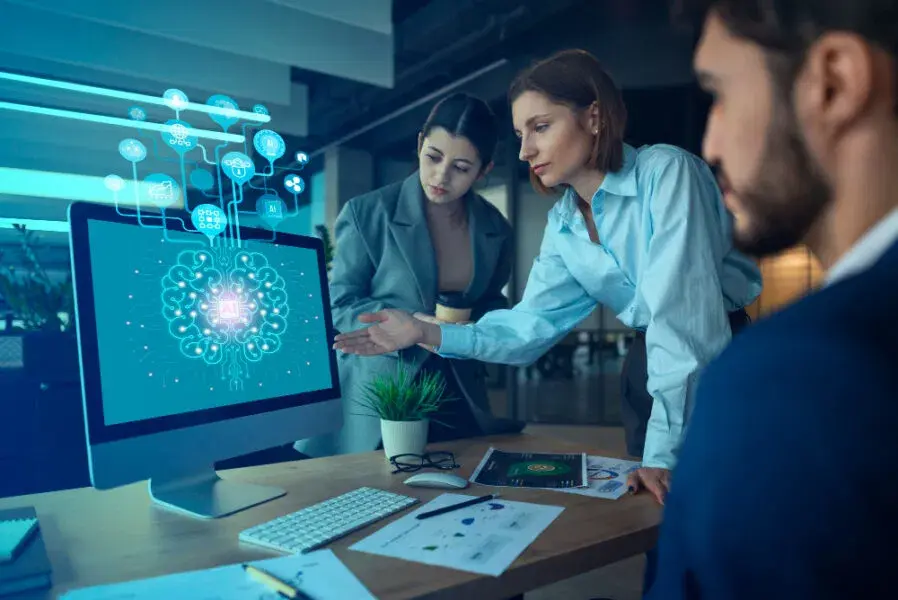
Probability theory forms the backbone of data analytics, providing essential tools for understanding uncertainty and making informed decisions based on data. As businesses and organizations increasingly rely on data-driven strategies, the principles of probability become crucial for interpreting results, predicting outcomes, and optimizing processes. This article explores the fundamentals of probability theory and its applications in data analytics, presenting concepts in an accessible manner.
Understanding Probability
At its core, probability quantifies uncertainty. It measures the likelihood of an event occurring, expressed as a number between 0 and 1. An event with a probability of 0 will never happen, while an event with a probability of 1 is certain to occur. Most events fall somewhere in between.
For instance, when flipping a fair coin, the probability of getting heads is 0.5, reflecting the equal likelihood of heads or tails. In more complex scenarios, probability can involve multiple events and their interactions, leading us to concepts such as joint probability and conditional probability.
Key Concepts in Probability
Random Variables: A random variable assigns a numerical value to each possible outcome of a random phenomenon. Random variables can be discrete (with specific values, like rolling a die) or continuous (with a range of values, like measuring height).
Probability Distributions: These functions describe how probabilities are distributed over the values of a random variable. Common distributions include the normal distribution, which is symmetrical and bell-shaped, and the binomial distribution, which applies to scenarios with two possible outcomes.
Bayes’ Theorem: This theorem provides a way to update probabilities based on new information. It plays a crucial role in many data analytics applications, particularly in machine learning and predictive modeling.
Central Limit Theorem: This fundamental theorem states that the sum of a large number of independent random variables tends toward a normal distribution, regardless of the original distribution. This principle allows analysts to make inferences about populations based on sample data.
Applications of Probability in Data Analytics
Probability theory finds a wide range of applications in data analytics, enabling analysts to derive insights and make predictions. Here are some key areas where probability plays a vital role:
1. Risk Assessment
Organizations use probability to assess risks associated with decisions or investments. By calculating the probability of various outcomes, businesses can make informed choices that balance potential gains against possible losses. For example, financial analysts may evaluate the risk of default on loans by analyzing historical data and using probability models to predict future behavior.
2. Predictive Analytics
In predictive analytics, probability helps forecast future events based on historical data. By leveraging statistical models, analysts can estimate the likelihood of outcomes. For instance, a retail company may use probability to predict customer purchasing behavior, helping them optimize inventory levels and marketing strategies.
3. A/B Testing
A/B testing is a method used to compare two versions of a product or service to determine which performs better. Probability theory underpins this process by allowing analysts to assess the significance of observed differences. By calculating p-values, analysts can determine whether the results are due to chance or represent a meaningful difference in performance.
4. Machine Learning
Probability is integral to many machine learning algorithms. For example, Bayesian networks utilize probability to model relationships between variables, while decision trees use probabilistic splitting to improve prediction accuracy. Understanding these principles enables data scientists to create more robust models and improve their performance.
5. Quality Control
In manufacturing and production, probability plays a key role in quality control. Statistical process control (SPC) techniques use probability distributions to monitor production processes, helping organizations identify deviations from expected outcomes. By applying these techniques, businesses can maintain high-quality standards and reduce waste.
6. Sports Analytics
In sports, probability is used to analyze player performance, team strategies, and game outcomes. Analysts calculate the probability of various events, such as a player scoring or a team winning, based on historical data. This information can guide coaching decisions and improve team performance.
Conclusion
Probability theory is a foundational element of data analytics, providing essential tools for understanding and managing uncertainty. By grasping the key concepts of probability and their applications, analysts can enhance their decision-making capabilities and drive better outcomes for their organizations. As data continues to grow in importance, the role of probability in analytics will only become more significant, making it a valuable area of study for anyone involved in data-driven decision-making.
Whether you're in finance, marketing, manufacturing, or sports, a solid understanding of probability will empower you to navigate the complexities of data analytics and leverage insights to achieve success. For those looking to deepen their knowledge, a Data Analytics course in Lucknow, Delhi, Noida, and other cities in India can provide the necessary skills to excel in this field. These courses often cover probability theory alongside practical applications, equipping you with the tools needed for effective data analysis and informed decision-making.
Comentarios