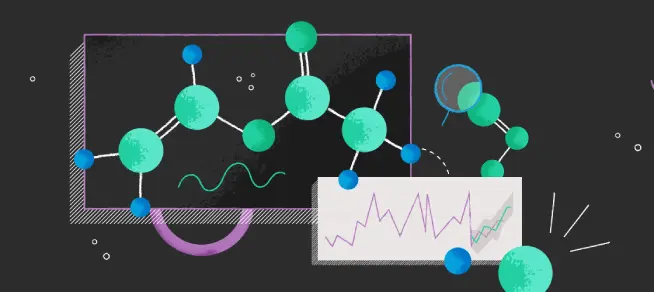
Organizations are bombarded with huge amounts of data in today's fast-moving digital world. Harnessing this data through predictive analytics can provide valuable insights, helping businesses make informed decisions and anticipate future trends. In this article, we’ll explore how data science powers predictive analytics, the steps involved in the process, and best practices to ensure success.
Understanding Predictive Analytics
Predictive analytics is a branch of advanced analytics that uses historical data, machine learning algorithms, and statistical techniques to forecast future outcomes. Organizations can anticipate behaviors, optimize processes, and improve decision-making by identifying patterns and trends.
Key Components of Predictive Analytics
Data Collection: The initial step is to collect relevant information from a variety of sources. This data can include customer interactions, sales figures, social media activity, and more.
Data Cleaning and Preparation: Raw data frequently includes errors, inconsistencies, and missing values. Data cleaning involves removing these discrepancies and preparing the dataset for analysis.
Exploratory Data Analysis (EDA): EDA helps identify patterns and relationships within the data. This step involves visualizing data and calculating descriptive statistics to understand its structure.
Model Selection: Various algorithms are available for predictive modeling, including regression analysis, decision trees, and neural networks. The choice of model depends on the specific problem and the nature of the data.
Model Training: During this phase, the selected model is trained using historical data. The model learns to recognize patterns and make predictions based on the provided input.
Model Evaluation: After training, the model’s performance is assessed using metrics like accuracy, precision, recall, and F1 score. This step ensures that the model generalizes well to new data.
Deployment and Monitoring: Once validated, the model is deployed in a real-world setting. Continuous monitoring is essential to maintain its performance and make necessary adjustments.
Steps to Implement Predictive Analytics
Step 1: Define the Problem
Before diving into data, clearly define the problem you want to solve. This may involve forecasting sales, predicting customer churn, or identifying potential fraud. A well-defined problem sets the foundation for the entire predictive analytics process.
Step 2: Gather and Prepare Data
Collect data from various sources, such as databases, APIs, and spreadsheets. Ensure that the data is relevant and comprehensive. Next, clean and preprocess the data to address any inconsistencies and fill in missing values.
Step 3: Conduct Exploratory Data Analysis
Perform EDA to visualize the data and uncover underlying patterns. Use tools like histograms, scatter plots, and correlation matrices to explore relationships among variables. This analysis can reveal important insights and guide the selection of the appropriate modeling techniques.
Step 4: Choose the Right Model
Selecting the right model is crucial for effective predictions. Consider the nature of the problem and the characteristics of your data. For instance, if you’re dealing with a continuous outcome variable, linear regression might be suitable. If you’re predicting categorical outcomes, decision trees or logistic regression could be more appropriate.
Step 5: Train the Model
Once you’ve selected a model, it’s time to train it using your dataset. Divide your data into training and testing sets to assess the model's performance. While training, the model learns to identify patterns and relationships in the data.
Step 6: Evaluate the Model
Assess the model's accuracy using various metrics. Common evaluation techniques include cross-validation and confusion matrices. These tools help ensure that the model performs well on unseen data and can be reliably used in practice.
Step 7: Deploy the Model
After successful evaluation, deploy the model to make predictions in real-time. This can be integrated into existing systems, allowing stakeholders to leverage insights as needed. Make sure to establish a monitoring system to track the model’s performance over time.
Best Practices for Successful Predictive Analytics
Collaborate Across Departments: Involve stakeholders from different departments, such as marketing, sales, and IT. Their insights can enhance the predictive model and ensure its alignment with business goals.
Prioritize Data Quality: Ensure that the data used is accurate, complete, and relevant. Poor data quality can lead to misleading results and ineffective predictions.
Iterate and Improve: Predictive analytics is not a one-time effort. Continuously refine your models based on new data and changing business needs. Regular updates help maintain the model’s relevance and accuracy.
Communicate Insights Effectively: Present your findings clearly and understandably. Use visualizations to make complex data more accessible and actionable for decision-makers.
Stay Ethical: Consider the ethical implications of your predictions. Ensure that your data practices comply with regulations and prioritize transparency and fairness.
Conclusion
Predictive analytics, powered by data science, offers immense potential for organizations looking to gain a competitive edge. By understanding the steps involved and adhering to best practices, businesses can harness their data to make informed predictions, optimize operations, and enhance customer experiences.
For those interested in further developing their skills, exploring a Data Science course in Delhi, Noida, Lucknow, Nagpur, and other cities in India can provide valuable knowledge in predictive analytics. As data continues to grow, so will the opportunities for predictive analytics, paving the way for smarter, data-driven decisions.
Comments