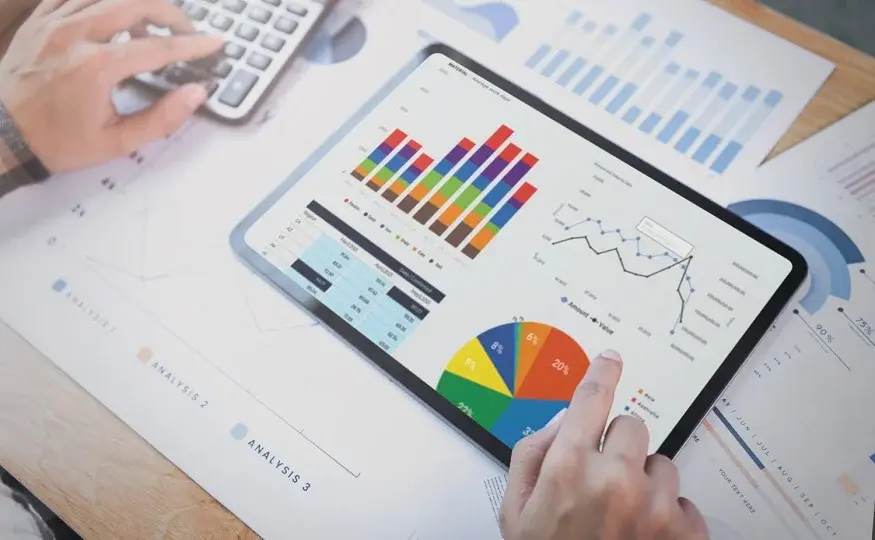
In the era of data-driven innovation, statistics serves as the cornerstone of data science. It transforms raw data into actionable insights, empowering professionals to make well-informed decisions. From identifying trends to predicting future outcomes, statistics bridges the gap between complex datasets and strategic decision-making.
This article delves into how statistics enhances decision-making in data science, exploring its key concepts, techniques, and real-world applications.
The Role of Statistics in Data Science
Statistics provides the tools to interpret data effectively, making it a critical component of data science. It enables professionals to:
Summarize DataDescriptive statistics help simplify large datasets by summarizing them into meaningful measures like mean, median, mode, and standard deviation. These summaries provide a snapshot of the data, making it easier to understand patterns and trends.
Draw ConclusionsInferential statistics facilitate decision-making by using sample data to infer properties about the population. Techniques like hypothesis testing and confidence intervals help ensure conclusions are reliable.
Identify RelationshipsStatistical models, such as correlation and regression analysis, uncover relationships between variables. For instance, regression helps predict outcomes based on input variables, aiding in strategic planning.
Core Statistical Techniques Used in Data Science
1. Hypothesis Testing
Hypothesis testing evaluates assumptions about datasets. For example, it can test whether a marketing strategy has significantly increased customer engagement or whether observed results occurred by chance.
2. Regression Analysis
Regression models are fundamental in predicting outcomes. Linear regression predicts continuous variables, such as sales forecasts, while logistic regression is used for binary outcomes like customer churn or fraud detection.
3. Probability Distributions
Understanding probability distributions, such as normal, binomial, or Poisson distributions, helps model data and assess uncertainties. These distributions play a significant role in predictive analytics.
4. Data Sampling
Sampling techniques ensure that data analysis is representative and unbiased. Random sampling, stratified sampling, and cluster sampling methods are commonly employed to gather meaningful data subsets.
5. Time Series Analysis
Time series analysis examines data points collected over time. It is widely used for forecasting trends, such as stock prices, energy demand, or website traffic.
How Statistics Drives Better Decision-Making
1. Enabling Data-Driven Insights
Statistics transforms raw data into actionable insights. For example, analyzing sales data can reveal seasonality patterns, helping businesses optimize inventory and marketing strategies.
2. Reducing Uncertainty
Statistical models quantify uncertainties, allowing decision-makers to evaluate risks and opportunities accurately. For instance, predictive models can estimate customer lifetime value, guiding investment decisions.
3. Enhancing Predictive Capabilities
Predictive analytics, powered by statistics, anticipates future outcomes. This capability is crucial for industries like healthcare (predicting disease outbreaks) and finance (forecasting market trends).
4. Supporting Experimental Designs
Statistical methods like A/B testing enable businesses to experiment with different strategies. By comparing outcomes, they can determine the most effective approach to achieve desired results.
Applications of Statistics in Data Science
1. Healthcare
Statistics helps in analyzing patient data to predict diseases, optimize treatments, and improve healthcare delivery. For instance, regression models predict the likelihood of developing chronic illnesses.
2. Marketing and Sales
Businesses leverage statistical insights to segment customers, predict buying behavior, and optimize campaigns. Clustering algorithms, rooted in statistical methods, group customers based on shared characteristics.
3. Finance
In finance, statistics aids in risk assessment, fraud detection, and portfolio management. Techniques like Monte Carlo simulations model investment outcomes under various scenarios.
4. Manufacturing
Statistical quality control ensures products meet standards. Predictive models help reduce defects and improve production efficiency.
5. Technology
Technology companies use statistics to optimize algorithms, personalize user experiences, and predict user behaviors. For example, recommendation systems rely on statistical models to suggest relevant content.
The Future of Statistics in Data Science
As data grows in volume and complexity, the importance of statistics in data science continues to expand. Advanced techniques like Bayesian statistics and machine learning integrate statistical principles to build more sophisticated models. These advancements enable organizations to extract deeper insights and make data-driven decisions with greater precision.
Emerging areas such as artificial intelligence, big data, and IoT also rely heavily on statistical methods. As these fields evolve, professionals with strong statistical expertise will remain in high demand.
Conclusion
Statistics is not just a tool; it is a critical enabler of decision-making in data science. By leveraging statistical techniques, organizations can navigate uncertainty, uncover meaningful patterns, and predict future trends. From healthcare to finance, the applications of statistics are vast and transformative.
Mastering statistics equips data professionals with the skills needed to turn data into actionable strategies, ensuring businesses stay competitive in a rapidly evolving landscape. As a fundamental part of any Data Science course in Patna, Nagpur, Mumbai, Pune, and other cities in India, statistics plays a pivotal role in shaping the analytical mindset required to tackle real-world challenges.
In essence, statistics is the backbone of data science, driving innovation and informed decision-making.
Comments