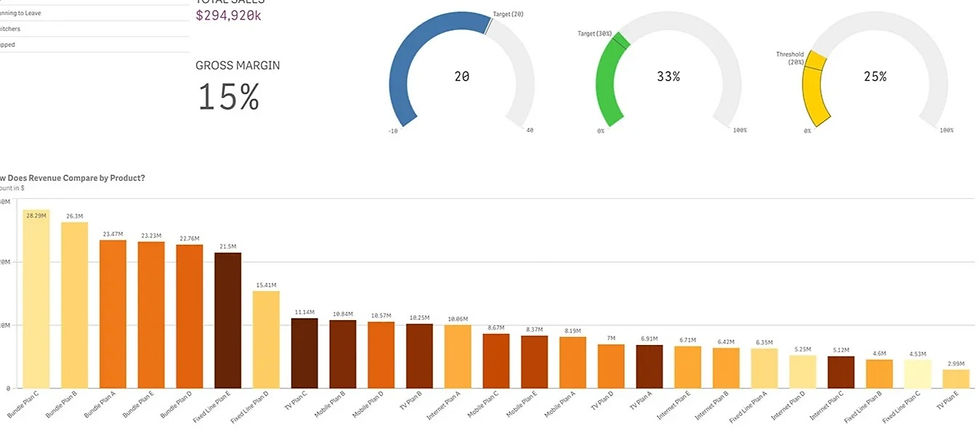
In today's data-driven world, effective analysis and interpretation of data are critical for informed decision-making. However, traditional data analysis methods often fail to fully engage users, resulting in missed insights and opportunities. This article explores the concept of "Data Dialogues" and how they foster user participation in analytics through the EVAL framework.
Introduction to Data Dialogues
Data Dialogues are interactive sessions or processes where users actively engage with data to derive insights and make decisions. Unlike traditional methods that rely on passive consumption of pre-generated reports or dashboards, Data Dialogues encourage active participation and collaboration between users and analysts.
The EVAL Framework
EVAL, a structured approach to data analysis and decision-making, comprises four key steps:
Evaluate: Users define the problem or question they wish to address, clarifying objectives, identifying relevant data sources, and formulating hypotheses.
Visualize: Once the problem is defined, users visualize data using charts, graphs, or other visualizations to explore patterns, trends, and outliers.
Act: Users take action based on their findings, which may involve making decisions, developing strategies, or implementing changes informed by insights gained from the data.
Learn: Users reflect on their actions and outcomes, incorporating learnings into future analyses to continually refine their analytical skills and decision-making.
Benefits of Data Dialogues with EVAL
Implementing Data Dialogues with the EVAL framework offers several advantages:
Increased User Engagement: Active involvement in the data analysis process increases user engagement and ownership of insights and decisions.
Enhanced Insights: Iterative exploration and discussion of data facilitate deeper insights and a comprehensive understanding of complex problems.
Improved Decision-Making: Direct interaction with data enables users to visualize findings, leading to more informed and evidence-based decision-making.
Iterative Learning: The iterative nature of the EVAL framework promotes continuous learning and improvement, refining analytical skills and approaches over time.
Case Study: Implementing Data Dialogues
Consider a hypothetical case study:
Scenario: A retail company aims to optimize its product pricing strategy for maximum profitability.
Evaluate: Users define the problem as optimizing product pricing for profitability, identifying relevant data sources like sales data, customer demographics, and competitor pricing.
Visualize: Users visualize sales data using charts and graphs to identify trends in product sales and customer purchasing behavior.
Act: Based on analysis, users develop a pricing strategy considering factors such as product demand, competitor prices, and customer preferences.
Learn: After implementing the new pricing strategy, users monitor sales performance, gather feedback, and incorporate learnings into future analyses for continual refinement.
Conclusion
Data Dialogues with the EVAL framework, along with comprehensive Data Analytics Training Course in Lucknow, Gwalior, Delhi, Noida, and all locations in India, offer a structured and collaborative approach to data analysis and decision-making. By fostering user participation and engagement, Data Dialogues empower organizations to derive deeper insights, make informed decisions, and drive continuous improvement. Implementing Data Dialogues requires a cultural shift towards valuing data literacy and fostering a collaborative data-driven mindset, but the benefits justify the effort.
Comments