Data Science 2025: Emerging Trends You Need to Know
- k86874248
- Feb 14
- 4 min read
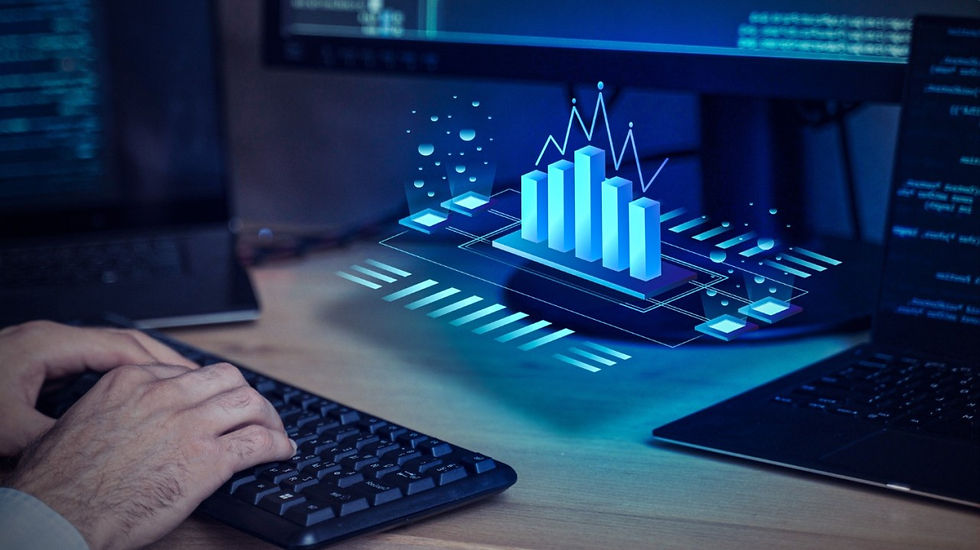
Data science is rapidly evolving, and staying ahead of the curve is crucial for anyone working in the field. As we look toward 2025, several key trends are set to shape the data science landscape. These trends will not only impact how data is analyzed but also how data professionals interact with technology. This article explores the emerging trends in data science that are set to define the future of the industry.
1. AI and Machine Learning Advancements
Artificial Intelligence (AI) and Machine Learning (ML) have been pivotal to the growth of data science, and this trend is expected to intensify in 2025. AI and ML technologies are becoming increasingly sophisticated, enabling businesses to automate decision-making processes and analyze vast amounts of data with greater accuracy. These technologies will play a larger role in predictive analytics, customer personalization, and business optimization.
Why it matters: Professionals in data science will need to refine their knowledge of AI and ML algorithms to remain relevant. As automation and AI-driven decision-making increase, data scientists will have the opportunity to focus more on higher-level tasks, such as model development and strategy.
2. Automation and Augmented Analytics
The automation of data science workflows is one of the most significant trends in the industry. Augmented analytics powered by AI will make it easier for non-experts to analyze data and generate insights. Tools that automate tasks like data cleaning, feature selection, and model tuning will continue to reduce the time and effort required to work with data.
Why it matters: With automated tools handling repetitive tasks, data scientists will have more time to focus on complex problem-solving and advanced analytics. Embracing these automated tools will be crucial for improving workflow efficiency and accelerating decision-making.
3. Explainable AI (XAI)
As AI models become more complex, the demand for transparency has increased. Explainable AI (XAI) focuses on making AI decision-making processes more understandable to humans. This trend is essential for gaining trust in AI systems, particularly in sectors like healthcare, finance, and law, where AI-driven decisions must be transparent and accountable.
Why it matters: Data scientists will need to understand and implement explainable AI techniques to build trust in their models. This shift will help ensure that AI applications are ethical, compliant with regulations, and accepted by stakeholders.
4. The Power of Data Visualization
Data visualization continues to evolve, enabling professionals to present complex data insights in a more intuitive and accessible manner. In 2025, the use of advanced visualization tools will become more widespread. These tools will allow data scientists to create interactive dashboards, real-time analytics, and compelling visual stories from data.
Why it matters: As organizations rely on data to inform decisions, clear and effective data visualization will become a key skill for data scientists. Professionals who can translate raw data into actionable insights through interactive visuals will stand out in the industry.
5. Data Privacy and Ethics
With the rise in data collection, data privacy and ethics are becoming more significant concerns. As data scientists, it is essential to understand the legal frameworks surrounding data protection, such as the General Data Protection Regulation (GDPR) and California Consumer Privacy Act (CCPA). Ensuring that data is collected, processed, and analyzed ethically will become even more important as data use continues to expand.
Why it matters: Data scientists will need to be vigilant in ensuring that their work complies
with privacy laws and ethical standards. This includes developing AI and ML models that do not inadvertently discriminate against certain groups and maintaining transparency in data usage.
6. Edge Computing and IoT
Edge computing is rapidly becoming an integral part of data science, especially with the rise of Internet of Things (IoT) devices. Instead of sending all data to the cloud for processing, edge computing processes data closer to where it is generated, reducing latency and improving speed.
Why it matters: The growing volume of real-time data from IoT devices presents new opportunities for data scientists. By working with edge computing systems, professionals can develop models that analyze data in real time, enabling faster decision-making in fields like autonomous vehicles, healthcare, and smart cities.
7. Cloud Platforms and Data Management
Cloud computing has revolutionized how data is stored, managed, and analyzed. In 2025, cloud platforms will continue to evolve, offering greater flexibility and integration with AI, machine learning, and real-time data processing tools. These platforms will make it easier for organizations to scale their data infrastructure without the need for expensive on-site equipment.
Why it matters: Cloud platforms are becoming a cornerstone of data science workflows. Data scientists who are proficient in cloud-based tools and data management systems will be better positioned to support the growing data needs of organizations.
8. Natural Language Processing (NLP) Innovations
Natural Language Processing (NLP) continues to make strides, enabling machines to understand, interpret, and generate human language. By 2025, NLP models will become more sophisticated, allowing for better sentiment analysis, chatbots, voice assistants, and automated content generation.
Why it matters: As NLP technology advances, data scientists will increasingly work with text and language data. The ability to build and optimize NLP models will be an important skill for data professionals who work with large volumes of unstructured data.
9. Quantum Computing
Although still in its infancy, quantum computing has the potential to revolutionize data science by solving complex problems that are impossible for classical computers to handle. By leveraging the principles of quantum mechanics, quantum computers could significantly speed up the process of data analysis and optimization.
Why it matters: While quantum computing is not yet widespread, data scientists who explore this emerging field will be well-positioned to take advantage of its future potential. Understanding quantum algorithms and their applications will be a valuable asset for professionals in the field.
Conclusion
To stay ahead in the ever-evolving world of data science, professionals must continuously adapt to emerging trends like AI, machine learning, and automation. Gaining expertise in these areas, along with skills in data visualization and NLP, is essential. To stay updated with the latest tools and technologies, Data Analytics Training in Noida, Delhi, Lucknow, Nagpur, and other cities in India can provide the knowledge and hands-on experience needed to succeed in this dynamic field. Visit local institutes for more information on courses that will help you enhance your career.
Comentarios