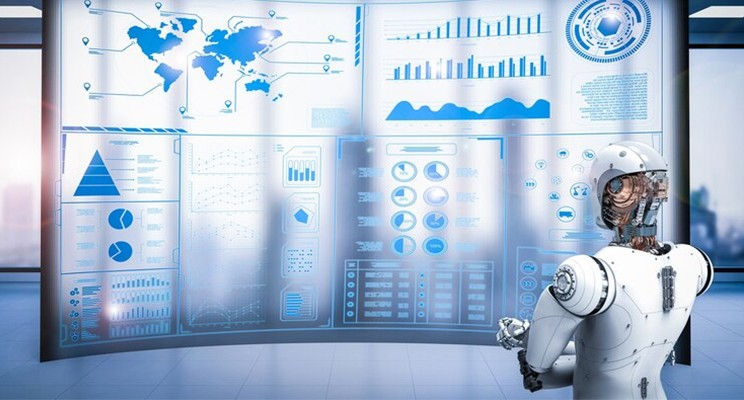
In today’s digital world, data is more than just numbers; it's a valuable asset, powering decision-making and innovation across industries. As data accumulates from every digital interaction—be it social media, online purchases, or sensor readings from IoT devices—businesses are learning how to analyze massive datasets to gain valuable insights. This process, known as Big Data Analytics, turns overwhelming amounts of data into actionable intelligence.
What is Big Data Analytics?
Big Data Analytics involves collecting, organizing, and analyzing large datasets to discover patterns, trends, and insights that inform critical decisions. These datasets, commonly called “big data,” are defined by their high volume, velocity, and variety. Traditional data processing tools often struggle to handle the scale and complexity of such data, which is where specialized analytics tools come in. Through techniques like data mining, machine learning, and statistical analysis, big data analytics extracts meaning from complex datasets.
The Characteristics of Big Data
Big data is typically defined by three core characteristics:
Volume: Big data refers to vast amounts of information generated from various sources, from social media posts to digital transactions. This data is so extensive that traditional storage methods are inadequate, requiring advanced systems for processing.
Velocity: Data is generated at unprecedented speeds, often in real time, which demands high-speed processing and response systems.
Variety: Big data comes in diverse formats, including structured data (like databases), semi-structured data (like XML files), and unstructured data (like videos and text documents). This diversity adds complexity to analytics but also provides rich insights when processed effectively.
Why Big Data Analytics Matters
Big Data Analytics enables organizations to gain a competitive edge by uncovering insights that would otherwise remain hidden. Here’s how it drives value across industries:
Enhanced Decision-Making: With access to real-time insights, businesses can make decisions quickly, backed by data. For example, in finance, real-time data analysis helps detect fraudulent transactions instantly, protecting customers and reducing risks for financial institutions.
Customer Personalization: Big data allows companies to gain deeper insights into customer preferences and customize their products or services accordingly.
By analyzing purchase histories, social media interactions, and browsing patterns, companies can deliver personalized experiences that improve customer satisfaction and loyalty.
Operational Efficiency: Big data analytics can streamline operations by identifying inefficiencies in production, distribution, or resource allocation. In manufacturing, for example, predictive maintenance helps identify machinery likely to fail, reducing downtime and saving costs.
Market Forecasting: Analyzing market trends and consumer behavior data empowers businesses to forecast demand and adjust strategies. This insight is especially useful in retail, where inventory management directly affects profitability.
Tools and Techniques in Big Data Analytics
Big data analytics relies on advanced tools and methodologies designed to handle massive datasets. Common techniques include:
Data Mining: Extracting valuable information from large datasets by identifying patterns.
Machine Learning: Utilizing algorithms to enable computers to learn from data and make predictions.
Natural Language Processing (NLP): Analyzing and interpreting human language data from sources like social media and customer reviews.
Some popular tools in big data analytics are Apache Hadoop, Spark, and Tableau. These tools make it easier to process, analyze, and visualize large datasets, helping businesses make data-driven decisions efficiently.
Challenges in Big Data Analytics
While big data analytics offers incredible benefits, it also presents challenges:
Data Privacy and Security: With increasing data volumes, protecting sensitive information is crucial. Ensuring data privacy and compliance with regulations like GDPR necessitates strong security protocols.
Data Quality: Inaccurate or unreliable data can result in flawed insights. Companies need to ensure data accuracy and consistency across sources.
High Costs: Implementing big data solutions often requires significant investment in infrastructure and skilled personnel. Organizations must balance these costs with the potential benefits.
The Future of Big Data Analytics
Big data analytics is growing in importance as technology advances, reshaping how businesses operate. Innovations like AI and machine learning make data analysis faster and more accurate, allowing companies to process massive datasets in real time. As the volume of data expands, drawing actionable insights will be vital for organizations aiming to thrive in a data-driven world.
A Data Analytics course in Noida, Delhi, Lucknow, Nagpur, and other cities in India equips professionals with skills to transform raw data into strategic resources. By uncovering patterns and trends, data analytics enables better decision-making, enhances customer experiences, and boosts operational efficiency—essential for sustainable growth and relevance.
Comentarios