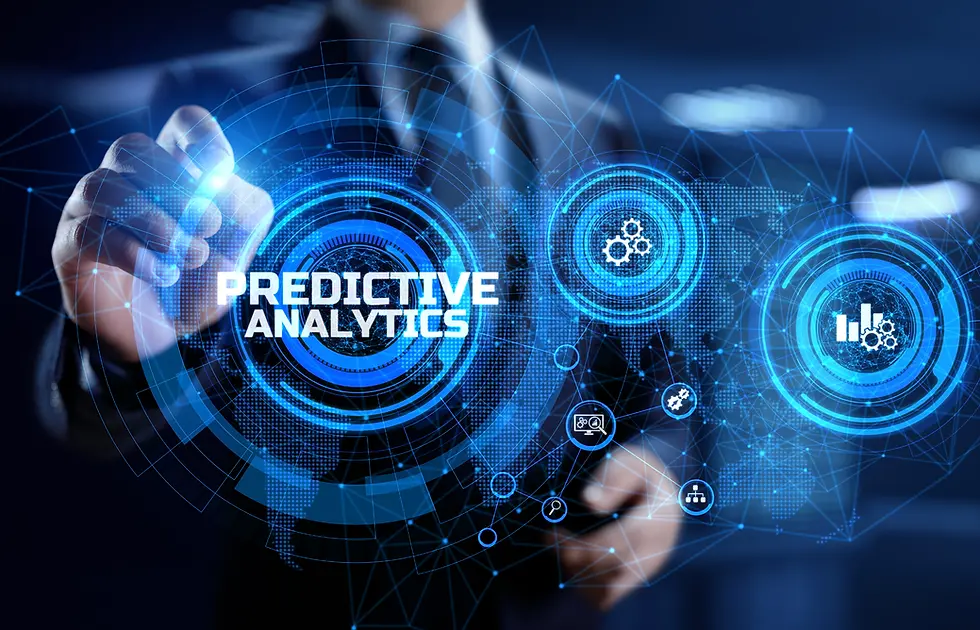
In today’s fast-paced digital landscape, businesses rely heavily on data-driven strategies to guide decision-making, uncover hidden patterns, and gain a competitive edge. Advanced data analytics and predictive modeling serve as powerful tools in this journey, enabling organizations to turn raw data into actionable insights. As technology progresses, these fields are becoming indispensable for achieving deeper understanding, identifying opportunities, and predicting future trends.
In this article, we'll explore the essentials of advanced data analytics and predictive modeling, discuss key techniques, and look at their applications in various industries. Whether you're a newcomer to data science or an experienced professional, this overview will provide useful insights into how these tools can shape business success.
Understanding Advanced Data Analytics
Data analytics involves the systematic examination of datasets to draw conclusions and make informed decisions. Advanced data analytics goes a step further by applying complex techniques and sophisticated tools, transforming traditional analytics into a more powerful and precise approach.
Advanced analytics encompasses several areas:
Descriptive Analytics – This first layer of analytics focuses on past and current data to provide insight into what has happened. It typically includes basic statistical techniques like mean, median, and standard deviation to summarize historical data.
Diagnostic Analytics – Diagnostic analytics goes beyond descriptive data to determine why something happened. It often involves hypothesis testing, data mining, and correlation analysis to identify root causes of trends or anomalies.
Predictive Analytics – Predictive analytics employs historical data to forecast future events or trends. By identifying patterns within the data, predictive analytics helps businesses anticipate outcomes and make proactive decisions.
Prescriptive Analytics – Prescriptive analytics takes predictive analytics a step further, offering recommendations for actions based on predictions. This includes optimization and simulation techniques, guiding organizations toward the best possible course of action.
Unpacking Predictive Modeling
Predictive modeling uses historical data to build mathematical models that can forecast future outcomes. It identifies relationships and patterns within the data that can predict future behavior or events. Predictive models are essential in sectors where foresight is crucial, such as finance, healthcare, retail, and marketing.
Key techniques used in predictive modeling include:
Regression Analysis: This technique identifies relationships between variables and predicts a continuous outcome, such as sales revenue or temperature.
Decision Trees: Decision trees split data into branches based on various conditions and are particularly useful for classification tasks, like determining whether a customer is likely to respond to a marketing campaign.
Random Forests: An extension of decision trees, random forests use multiple trees to improve predictive accuracy and prevent overfitting, which can occur with a single tree.
Neural Networks: Inspired by the human brain, neural networks are algorithms that recognize patterns. They are widely used in image and speech recognition, making them essential for modern AI applications.
Time Series Analysis: This technique predicts future values based on historical data collected over time intervals. It is particularly useful in stock market prediction, sales forecasting, and economic analysis.
Applications of Advanced Analytics and Predictive Modeling
Advanced analytics and predictive modeling have applications across various industries. Here’s a look at how they’re transforming some key sectors:
HealthcareIn healthcare, predictive modeling is revolutionizing patient care and operational efficiency. By analyzing patient data, predictive models can foresee potential health risks, enabling early intervention. For example, predictive analytics helps hospitals anticipate patient admission rates, optimize staffing, and reduce wait times, ultimately enhancing patient care.
FinanceThe finance sector relies heavily on predictive modeling for risk assessment, fraud detection, and portfolio management. By analyzing transaction patterns, financial institutions can identify fraudulent activities in real time. Predictive models also help in credit scoring, assessing an individual’s creditworthiness based on historical data.
RetailRetailers use predictive analytics to understand customer behavior, personalize marketing efforts, and optimize inventory. By analyzing purchasing patterns, retailers can predict demand for specific products, ensuring they stock the right items at the right time. This not only boosts sales but also enhances customer satisfaction by providing personalized experiences.
ManufacturingIn manufacturing, predictive maintenance is a game-changer. By analyzing machine data, predictive models can foresee equipment failures, allowing companies to conduct timely maintenance and reduce downtime. This not only saves costs but also improves productivity and safety.
MarketingMarketers leverage predictive modeling to segment audiences, forecast campaign outcomes, and personalize customer interactions. By understanding what appeals to each customer segment, companies can deliver more effective campaigns and improve conversion rates.
The Benefits and Challenges of Implementing Advanced Analytics
Benefits
Implementing advanced analytics offers several advantages:
Improved Decision-Making: By providing actionable insights, advanced analytics enables data-driven decisions that are more informed and strategic.
Enhanced Customer Experience: With predictive insights, businesses can personalize experiences, meeting customer needs more effectively.
Increased Efficiency: Through process optimization and predictive maintenance, companies can reduce costs and boost operational efficiency.
Competitive Advantage: By leveraging analytics, organizations can stay ahead of industry trends, positioning themselves as leaders in their fields.
ChallengesDespite the benefits, implementing advanced analytics can pose challenges:
Data Quality: Analytics are only as good as the data they rely on. Inaccurate or incomplete data can lead to flawed insights.
Complexity and Cost: Advanced analytics systems often require significant investment and specialized skills, which can be a barrier for some organizations.
Privacy Concerns: Handling vast amounts of personal data raises privacy issues. Organizations must comply with regulations and ensure data security.
Future Trends in Data Analytics and Predictive Modeling
As technology continues to evolve, so do the possibilities in data analytics and predictive modeling. Emerging trends in the field include:
AI and Machine Learning Integration: Machine learning is becoming integral to predictive modeling, allowing for more accurate and scalable solutions.
Real-Time Analytics: As businesses demand faster insights, real-time analytics is becoming more prominent. This trend enables organizations to act immediately on new information.
Explainable AI: With growing demand for transparency, explainable AI is gaining attention. This approach aims to make AI models understandable, ensuring trust and accountability.
Conclusion
Advanced data analytics and predictive modeling hold transformative potential for businesses across industries. By harnessing these tools, organizations can gain valuable insights, anticipate trends, and make proactive decisions that drive success. For those looking to enter this field or deepen their expertise, pursuing a Data Analytics Training Course in Delhi, Noida, Lucknow, Nagpur, and more cities in India can be an ideal step. As data-driven strategies become central to modern business, those who embrace advanced analytics will be better positioned to thrive in a competitive, ever-evolving l
Comments